Abstract
Genomic biomarkers for the detection of drug-induced liver injury (DILI) from blood are urgently needed for monitoring drug safety. We used a unique data set as part of the Food and Drug Administration led MicroArray Quality Control Phase-II (MAQC-II) project consisting of gene expression data from the two tissues (blood and liver) to test cross-tissue predictability of genomic indicators to a form of chemically induced liver injury. We then use the genomic indicators from the blood as biomarkers for prediction of acetaminophen-induced liver injury and show that the cross-tissue predictability of a response to the pharmaceutical agent (accuracy as high as 92.1%) is better than, or at least comparable to, that of non-therapeutic compounds. We provide a database of gene expression for the highly informative predictors, which brings biological context to the possible mechanisms involved in DILI. Pathway-based predictors were associated with inflammation, angiogenesis, Toll-like receptor signaling, apoptosis, and mitochondrial damage. The results show for the first time and support the hypothesis that genomic indicators in the blood can serve as potential diagnostic biomarkers predictive of DILI.
This is a preview of subscription content, access via your institution
Access options
Subscribe to this journal
Receive 6 print issues and online access
$259.00 per year
only $43.17 per issue
Buy this article
- Purchase on Springer Link
- Instant access to full article PDF
Prices may be subject to local taxes which are calculated during checkout




Similar content being viewed by others
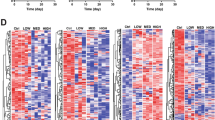
Accession codes
References
Bushel PR, Heinloth AN, Li J, Huang L, Chou JW, Boorman GA et al. Blood gene expression signatures predict exposure levels. Proc Natl Acad Sci USA 2007; 104: 18211–18216.
Lobenhofer EK, Auman JT, Blackshear PE, Boorman GA, Bushel PR, Cunningham ML et al. Gene expression response in target organ and whole blood varies as a function of target organ injury phenotype. Genome Biol 2008; 9: R100.
Wang K, Zhang S, Marzolf B, Troisch P, Brightman A, Hu Z et al. Circulating microRNAs, potential biomarkers for drug-induced liver injury. Proc Natl Acad Sci USA 2009; 106: 4402–4407.
Waters M, Stasiewicz S, Merrick BA, Tomer K, Bushel P, Paules R et al. CEBS—chemical effects in biological systems: a public data repository integrating study design and toxicity data with microarray and proteomics data. Nucleic Acids Res 2008; 36 (Database issue): D892–D900.
Hubbell E, Liu WM, Mei R . Robust estimators for expression analysis. Bioinformatics (Oxford, England) 2002; 18: 1585–1592.
Huang L, Heinloth AN, Zeng ZB, Paules RS, Bushel PR . Genes related to apoptosis predict necrosis of the liver as a phenotype observed in rats exposed to a compendium of hepatotoxicants. BMC Genomics 2008; 9: 288.
Bushel PR, Hamadeh H, Bennett L, Sieber S, Martin K, Nuwaysir EF et al. MAPS: a microarray project system for gene expression experiment information and data validation. Bioinformatics (Oxford, England) 2001; 17: 564–565.
Tong W, Harris S, Cao X, Fang H, Shi L, Sun H et al. Development of public toxicogenomics software for microarray data management and analysis. Mutat Res 2004; 549: 241–253.
Boorman GA, Haseman JK, Waters MD, Hardisty JF, Sills RC . Quality review procedures necessary for rodent pathology databases and toxicogenomic studies: the National Toxicology Program experience. Toxicol Pathol 2002; 30: 88–92.
Nikolsky Y, Kirillov E, Zuev R, Rakhmatulin E, Nikolskaya T . Functional analysis of OMICs data and small molecule compounds in an integrated ‘knowledge-based’ platform. Methods Mol Biol (Clifton, NJ) 2009; 563: 177–196.
Liaw A, Wiener M . Classification and regression by randomForest. R News 2002; 2: 18–22.
Chou JW, Bushel PR . Discernment of possible mechanisms of hepatotoxicity via biological processes over-represented by co-expressed genes. BMC Genomics 2009; 10: 272.
Chou JW, Zhou T, Kaufmann WK, Paules RS, Bushel PR . Extracting gene expression patterns and identifying co-expressed genes from microarray data reveals biologically responsive processes. BMC Bioinformatics 2007; 8: 427.
Hosack DA, Dennis Jr G, Sherman BT, Lane HC, Lempicki RA . Identifying biological themes within lists of genes with EASE. Genome Biol 2003; 4: R70.
Zheng Q, Wang XJ . GOEAST: a web-based software toolkit for Gene Ontology enrichment analysis. Nucleic Acids Res 2008; 36 (Web Server issue): W358–W363.
Alexa A, Rahnenfuhrer J, Lengauer T . Improved scoring of functional groups from gene expression data by decorrelating GO graph structure. Bioinformatics (Oxford, England) 2006; 22: 1600–1607.
Benjamini Y, Yekutieli D . The control of the false discovery rate in multiple testing under dependency. Ann Stat 2001; 29: 1165–1188.
Casarett LJ, Doull J, Klaassen CD . Casarett and Doull's Toxicology: The Basic Science of Poisons, 6th edn. McGraw-Hill Medical Pub. Division: New York, 2001, xix, 1236 pp.
Neff SB, Neff TA, Kunkel SL, Hogaboam CM . Alterations in cytokine/chemokine expression during organ-to-organ communication established via acetaminophen-induced toxicity. Exp Mol Pathol 2003; 75: 187–193.
Jaeschke H, Hasegawa T . Role of neutrophils in acute inflammatory liver injury. Liver Int 2006; 26: 912–919.
Ramaiah SK, Jaeschke H . Role of neutrophils in the pathogenesis of acute inflammatory liver injury. Toxicol Pathol 2007; 35: 757–766.
Jaeschke H, Gores GJ, Cederbaum AI, Hinson JA, Pessayre D, Lemasters JJ . Mechanisms of hepatotoxicity. Toxicol Sci 2002; 65: 166–176.
Kaplowitz N . Biochemical and cellular mechanisms of toxic liver injury. Semin Liver Dis 2002; 22: 137–144.
Lee WM . Drug-induced hepatotoxicity. N Engl J Med 2003; 349: 474–485.
Yohe HC, O’Hara KA, Hunt JA, Kitzmiller TJ, Wood SG, Bement JL et al. Involvement of Toll-like receptor 4 in acetaminophen hepatotoxicity. Am J Physiol Gastrointest Liver Physiol 2006; 290: G1269–G1279.
Ozer J, Ratner M, Shaw M, Bailey W, Schomaker S . The current state of serum biomarkers of hepatotoxicity. Toxicology 2008; 245: 194–205.
Kaplowitz N, DeLeve LD . Drug-Induced Liver Disease, 2nd edn. Informa Healthcare: New York, 2007, xv, 808 pp.
Kudo Y, Ochi T, Shimada H, Ogawa S, Shinjo K . Utility of plasma circulating mRNA as a marker to detect hepatic injury. J Vet Med Sci 2008; 70: 993–995.
Nakken KE, Nygard S, Haaland TK, Berge KE, Odegaard A, Labori KJ et al. Gene expression profiles reflect sclerosing cholangitis activity in abcb4 (−/−) mice. Scand J Gastroenterol 2009; 44: 211–218.
Zhai Y, Shen XD, Gao F, Zhao A, Freitas MC, Lassman C et al. CXCL10 regulates liver innate immune response against ischemia and reperfusion injury. Hepatology 2008; 47: 207–214.
Yoneyama H, Kai Y, Koyama J, Suzuki K, Kawachi H, Narumi S et al. Neutralization of CXCL10 accelerates liver regeneration in carbon tetrachloride-induced acute liver injury. Med Mol Morphol 2007; 40: 191–197.
Ikemoto M, Murayama H, Itoh H, Totani M, Fujita M . Intrinsic function of S100A8/A9 complex as an anti-inflammatory protein in liver injury induced by lipopolysaccharide in rats. Clin Chim Acta 2007; 376: 197–204.
Tester AM, Cox JH, Connor AR, Starr AE, Dean RA, Puente XS et al. LPS responsiveness and neutrophil chemotaxis in vivo require PMN MMP-8 activity. PLoS ONE 2007; 2: e312.
Rosas IO, Richards TJ, Konishi K, Zhang Y, Gibson K, Lokshin AE et al. MMP1 and MMP7 as potential peripheral blood biomarkers in idiopathic pulmonary fibrosis. PLoS Med 2008; 5: e93.
Minami K, Saito T, Narahara M, Tomita H, Kato H, Sugiyama H et al. Relationship between hepatic gene expression profiles and hepatotoxicity in five typical hepatotoxicant-administered rats. Toxicol Sci 2005; 87: 296–305.
Burczynski ME, Dorner AJ . Transcriptional profiling of peripheral blood cells in clinical pharmacogenomic studies. Pharmacogenomics 2006; 7: 187–202.
Gangadharan B, Antrobus R, Dwek RA, Zitzmann N . Novel serum biomarker candidates for liver fibrosis in hepatitis C patients. Clin Chem 2007; 53: 1792–1799.
Miller T, Knapton A, Adeyemo O, Noory L, Weaver J, Hnig J . Cytochrome c: a non-invasive biomarker of drug-induced liver injury. J Appl Toxicol 2008; 28: 815–828.
Xu JJ, Henstock PV, Dunn MC, Smith AR, Chabot JR, de Graaf D . Cellular imaging predictions of clinical drug-induced liver injury. Toxicol Sci 2008; 105: 97–105.
Merrick BA, Bruno ME, Madenspacher JH, Wetmore BA, Foley J, Pieper R et al. Alterations in the rat serum proteome during liver injury from acetaminophen exposure. J Pharmacol Exp Ther 2006; 318: 792–802.
Amacher DE, Adler R, Herath A, Townsend RR . Use of proteomic methods to identify serum biomarkers associated with rat liver toxicity or hypertrophy. Clin Chem 2005; 51: 1796–1803.
Merrick BA . The plasma proteome, adductome and idiosyncratic toxicity in toxicoproteomics research. Brief Funct Genomics Proteomic 2008; 7: 35–49.
Acknowledgements
We thank the National Center for Toxicogenomics at the National Institute of Environmental Health Sciences (NIEHS) for the hepatotoxicant compendium data. In addition, we thank the participants of MAQC-II for comments, feedback, and discussions on the topic of this paper during teleconferences and face-to-face project meetings. We also thank K Shockley, A Merrick, S Hester, B Ward, and D Mendrick for their critical review of the manuscript. JH acknowledge the support of the Oak Ridge Institute for Science and Education (ORISE) for the Post-graduate Research Program at the National Center for Toxicological Research (NCTR), US Food and Drug Administration (FDA). JH also acknowledges the support of the China State-funded Study Abroad Program that is organized by the China Scholarship Council (CSC). JH and XF both acknowledge the Chinese Key Technologies R&D Program (No. 2005CB23402) and the National Science Foundation of China (No. 30801556) for support to participate in the MAQC-II project at the NCTR/FDA. This research was supported, in part by, the Intramural Research Program of the NIH and NIEHS (Z01 ES102345-03). This document has been reviewed in accordance with the US FDA and Environmental Protection Agency (EPA) policies and is approved for publication. Approval does not signify that the contents necessarily reflect the position or opinions of the FDA or EPA nor does mention of trade names or commercial products constitute endorsement or recommendation for use. The findings, views, and conclusions in this report are those of the authors and do not necessarily represent or reflect the views of the FDA or EPA.
Author information
Authors and Affiliations
Corresponding author
Ethics declarations
Competing interests
The authors declare no conflict of interest.
Additional information
Supplementary Information accompanies the paper on the The Pharmacogenomics Journal website
Rights and permissions
About this article
Cite this article
Huang, J., Shi, W., Zhang, J. et al. Genomic indicators in the blood predict drug-induced liver injury. Pharmacogenomics J 10, 267–277 (2010). https://doi.org/10.1038/tpj.2010.33
Received:
Revised:
Accepted:
Published:
Issue Date:
DOI: https://doi.org/10.1038/tpj.2010.33
Keywords
This article is cited by
-
Metabolic network-based predictions of toxicant-induced metabolite changes in the laboratory rat
Scientific Reports (2018)
-
Chemically induced hepatotoxicity in human stem cell-induced hepatocytes compared with primary hepatocytes and HepG2
Cell Biology and Toxicology (2016)
-
Molecular mechanisms of hepatic apoptosis
Cell Death & Disease (2014)
-
The MicroArray Quality Control (MAQC)-II study of common practices for the development and validation of microarray-based predictive models
Nature Biotechnology (2010)